Artificial intelligence will determine tree line by space images
6 August 2019 г.
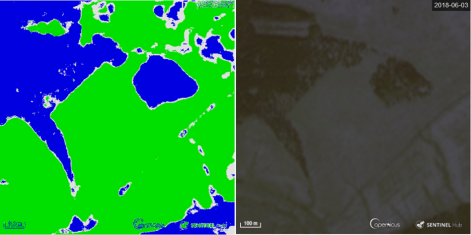
Multispectral satellite images can be used to study terrestrial vegetation and to determine the boundaries of various biomes, for example, the tree line. Data received from satellites are presented in the form of photographs. The information between an updated photograph and a traditional map often does not agree since maps are updated rarely. Manually analyzing images of thousands of square kilometers and drawing forests from photographs is an unrealistic task. Therefore, scientists decided to give this task to artificial intelligence.
A team of researchers from the Krasnoyarsk Institute of Biophysics and Institute of Computational Modeling of the Krasnoyarsk Science Center SB RAS have taught artificial intelligence to determine the type of vegetation in the areas and boundaries of biomes based on the Earth remote sensing data. Scientists have shown that the type of vegetation can be identified pixel by pixel using 12 spectral channels and a trained feedback algorithm. The program successfully recognizes and distinguishes between coniferous and deciduous forests, but so far has had problems with the meadow recognition.
The scientists trained the neural network using satellite images taken from May to September 2018. The data were provided from several areas of the Krasnoyarsk Region, wh ere the characteristic types of vegetation are well known. The neural network was trained on coniferous and mixed forests near Krasnoyarsk and on fields near the village Pogorelka. It learned to distinguish well the types of vegetation, as well as coniferous and deciduous forests by the appearance.
However, the algorithm still makes mistakes in recognizing meadows. Sometimes it recognizes there small areas as forests in the middle of the field. Scientists note that such a “defect” may be due to the presence of atypical plants there, with characteristics more appropriate for forests. The scientists are planning to increase the accuracy and improve recognition through additional training with a wider coverage of zones and data. The aim is also to reduce the number of channels used by the neural network.
“For a large area, whose images are to be viewed, a person can make mistakes due to tiredness or other factors. Our team suggested that a neural network should be faster and more accurate to perform such work. We took photographs of areas with precisely known types of vegetation in twelve spectral channels, and trained on them the neural network to recognize the boundaries of coniferous and deciduous forests and meadows. For each pixel of the image, the network received the input values of twelve spectral channels and it was trained to predict the type of vegetation.
Now we are trying to increase the recognition accuracy and the number of recognizable types of vegetation,” says Mikhail Saltykov, Candidate of Physical and Mathematical Sciences, junior research associate at the Institute of Biophysics SB RAS.
In the short-term, such a system will be able to track changes in forest areas. The neural network will automatically view and analyze images taken in different years and show how the tree line has moved during this period. With its help, it will be possible to track changes after fires, deforestation, advancement of the tree line due to climate warming and other factors.
The study was supported by the Russian Foundation for Basic Research and Russian Geographical Society.
Share: